Where did it come from and what is the supposed consensus? A good 16 minute clip on the answers can be found here at https://www.youtube.com/watch?v=ewJ6TI8ccAw
Category Posts by 4quarky
Fascinating series on CO2 and global warming
There is a fascinating series of posts on Dr. Robert Fagan’s site about CO2 that is definitely worth reading.
It can be found at dr-robert-fagan.com/shorter-posts/
Climate Models
There are an excellent series on climate models over at andymaypetrophysicist.com/category/climate-models
There are 7 issues on the models, that you will see as you scroll down the above link. They are: 1. What is a model, 2. Modeling Greenhouse Gases, 3. Solar Input, 4. Convection and atmospheric circulation, 5. Storminess, 6. WGII, and 7. WGIII
What is a Climate Model
Climate Model Bias 1: What is a Model?
Posted byAndy MayFebruary 28, 2024Posted inClimate models
By Andy May
There are three types of scientific models, as shown in figure 1. In this series of seven posts on climate model bias we are only concerned with two of them. The first are mathematical models that utilize well established physical, and chemical processes and principles to model some part of our reality, especially the climate and the economy. The second are conceptual models that utilize scientific hypotheses and assumptions to propose an idea of how something, such as the climate, works. Conceptual models are generally tested, and hopefully validated, by creating a mathematical model. The output from the mathematical model is compared to observations and if the output matches the observations closely, the model is validated. It isn’t proven, but it is shown to be useful, and the conceptual model gains credibility.
Figure 1. The three types of scientific models.
Models are useful when used to decompose some complex natural system, such as Earth’s climate, or some portion of the system, into its underlying components and drivers. Models can be used to try and determine which of the system components and drivers are the most important under various model scenarios.
Besides being used to predict the future, or a possible future, good models should also tell us what should not happen in the future. If these events do not occur, it adds support to the hypothesis. These are the tasks that the climate models created by the Coupled Model Intercomparison Project (CMIP)[1] are designed to do. The Intergovernmental Panel on Climate Change (IPCC)[2] analyzes the CMIP model results, along with other peer-reviewed research, and attempts to explain modern global warming in their reports. The most recent IPCC report is called AR6.[3]
In the context of climate change, especially regarding the AR6 IPCC[4] report, the term “model,” is often used as an abbreviation for a general circulation climate model.[5] Modern computer general circulation models have been around since the 1960s, and now are huge computer programs that can run for days or longer on powerful computers. However, climate modeling has been around for more than a century, well before computers were invented. Later in this report I will briefly discuss a 19th century greenhouse gas climate model developed and published by Svante Arrhenius.
Besides modeling climate change, AR6 contains descriptions of socio-economic models that attempt to predict the impact of selected climate changes on society and the economy. In a sense, AR6, just like the previous assessment reports, is a presentation of the results of the latest iteration of their scientific models of future climate and their models of the impact of possible future climates on humanity.
Introduction
Modern atmospheric general circulation computerized climate models were first introduced in the 1960s by Syukuro Manabe and colleagues.[6] These models, and their descendants can be useful, even though they are clearly oversimplifications of nature, and they are wrong[7] in many respects like all models.[8] It is a shame, but climate model results are often conflated with observations by the media and the public, when they are anything but.
I began writing scientific models of rocks[9] and programming them for computers in the 1970s and like all modelers of that era I was heavily influenced by George Box, the famous University of Wisconsin statistician. Box teaches us that all models are developed iteratively.[10] First we make assumptions and build a conceptual model about how some natural, economic, or other system works and what influences it, then we model some part of it, or the whole system. The model results are then compared to observations. There will typically be a difference between the model results and the observations, these differences are assumed to be due to model error since we necessarily assume our observations have no error, at least initially. We examine the errors, adjust the model parameters or the model assumptions, or both, and run it again, and again examine the errors. This “learning” process is the main benefit of models. Box tells us that good scientists must have the flexibility and courage to seek out, recognize, and exploit such errors, especially any errors in the conceptual model assumptions. Modeling nature is how we learn how nature works.
Box next advises us that “we should not fall in love with our models,” and “since all models are wrong the scientists cannot obtain a ‘correct’ one by excessive elaboration.” I used to explain this principle to other modelers more crudely by pointing out that if you polish a turd, it is still a turd. One must recognize when a model has gone as far as it can go. At some point it is done, more data, more elaborate programming, more complicated assumptions cannot save it. The benefit of the model is what you learned building it, not the model itself. When the inevitable endpoint is reached, you must trash the model and start over by building a new conceptual model. A new model will have a new set of assumptions based on the “learnings” from the old model, and other new data and observations gathered in the meantime.
Each IPCC report, since the first one was published in 1990,[11] is a single iteration of the same overall conceptual model. In this case, the “conceptual model” is the idea or hypothesis that humans control the climate (or perhaps just the rate of global warming) with our greenhouse gas emissions.[12] Various and more detailed computerized models are built to attempt to measure the impact of human emissions on Earth’s climate.
Another key assumption in the IPCC model is that climate change is dangerous, and, as a result, we must mitigate (reduce) fossil fuel use to reduce or prevent damage to society from climate change. Finally, they assume a key metric of this global climate change or warming is the climate sensitivity to human-caused increases in CO2. This sensitivity can be computed with models or using measurements of changes in atmospheric CO2 and global average surface temperature. The IPCC equates changes in global average surface temperature to “climate change.”
This climate sensitivity metric is often called “ECS,” which stands for equilibrium climate sensitivity to a doubling of CO2, often abbreviated as “2xCO2.”[13] Modern climate models, ever since those used for the famous Charney report in 1979,[14] except for AR6, have generated a range of ECS values from 1.5 to 4.5°C per 2xCO2. AR6 uses a rather unique and complex subjective model that results in a range of 2.5 to 4°C/2xCO2. More about this later in the report.
George Box warns modelers that:
“Just as the ability to devise simple but evocative models is the signature of the great scientist so overelaboration and overparameterization is often the mark of mediocrity.”[15]
Box, 1976
The Intergovernmental Panel on Climate Change or IPCC has published six major reports and numerous minor reports since 1990.[16] Here we will argue that they have spent more than thirty years polishing the turd to little effect. They have come up with more and more elaborate processes to try and save their hypothesis that human-generated greenhouse gases have caused recent climate changes and that the Sun and internal variations within Earth’s climate system have had little to no effect. As we will show, new climate science discoveries, since 1990, are not explained by the IPCC models, do not show up in the model output, and newly discovered climate processes, especially important ocean oscillations, are not incorporated into them.
Just one example. Eade, et al. report that the modern general circulation climate models used for the AR5 and AR6 reports[17] do not reproduce the important North Atlantic Ocean Oscillation (“NAO”). The NAO-like signal that the models produce in their simulation runs[18] is indistinguishable from random white noise. Eade, et al. report:
“This suggests that current climate models do not fully represent important aspects of the mechanism for low frequency variability of the NAO.”[19]
Eade, et al., 2022
All the models in AR6, both climate and socio-economic, have important model/observation mismatches. As time has gone on, the modelers and authors have continued to ignore new developments in climate science and climate change economics, as their “overelaboration and overparameterization” has become more extreme. As they make their models more elaborate, they progressively ignore more new data and discoveries to decrease their apparent “uncertainty” and increase their reported “confidence” that humans drive climate change. It is a false confidence that is due to the confirmation and reporting bias in both the models and the reports.
As I reviewed all six of the major IPCC reports, I became convinced that AR6 is the most biased of all of them.[20] In a major new book twelve colleagues and I, working under the Clintel[21] umbrella, examined AR6 and detailed considerable evidence of bias.
From the Epilog[22] of the Clintel book:
“AR6 states that “there has been negligible long-term influence from solar activity and volcanoes,”[23] and acknowledges no other natural influence on multidecadal climate change despite … recent discoveries, a true case of tunnel vision.”
“We were promised IPCC reports that would objectively report on the peer-reviewed scientific literature, yet we find numerous examples where important research was ignored. In Ross McKitrick’s chapter[24] on the “hot spot,” he lists many important papers that are not even mentioned in AR6. Marcel [Crok] gives examples where unreasonable emissions scenarios are used to frighten the public in his chapter on scenarios,[25] and examples of hiding good news in his chapter on extreme weather events.[26] Numerous other examples are documented in other chapters. These deliberate omissions and distortions of the truth do not speak well for the IPCC, reform of the institution is desperately needed.”
Crok and May, 2023
Confirmation[27] and reporting bias[28] are very common in AR6. We also find examples of the Dunning-Kruger effect,[29] in-group bias,[30] and anchoring bias.[31]
In 2010, the InterAcademy Council of the United Nations reviewed the processes and procedures of the IPCC and found many problems.[32] In particular, they criticized the subjective way that uncertainty is handled. They also criticized the obvious confirmation bias in the IPCC reports.[33] They pointed out that the Lead Authors too often leave out dissenting views or references to papers they disagree with. The Council recommended that alternative views should be mentioned and cited in the report. Even though these criticisms were voiced in 2010, I and my colleagues, found numerous examples of these problems in AR6, published eleven years later in 2021 and 2022.[34]
Although bias pervades AR6, this series will focus mainly on bias in the AR6 volume 1 (WGI) CMIP6[35] climate models that are used to predict future climate. However, we will also look at the models used to identify and quantify climate change impacts in volume 2 (WGII), and to compute the cost/benefit analysis of their recommended mitigation (fossil fuel reduction) measures in volume 3 (WGIII). As a former petrophysical modeler, I am aware how bias can sneak into a computer model, sometimes the modeler is aware he is introducing bias into the results, sometimes he is not. Bias exists in all models, since they are all built from assumptions and ideas (the “conceptual model”), but a good modeler will do his best to minimize it.
In the next six posts I will take you through some of the evidence of bias I found in the CMIP6 models and the AR6 report. A 30,000-foot look at the history of human-caused climate change modeling is given in part 2. Evidence that the IPCC has ignored possible solar influence on climate is presented in part 3. The IPCC ignores evidence that changes in convection and atmospheric circulation patterns in the oceans and atmosphere affect climate change on multidecadal times scales and this is examined in part 4.
Contrary to the common narrative, there is considerable evidence that storminess (extreme weather) was higher in the Little Ice Age, aka the “pre-industrial” (part 5). Next, we move on to examine bias in the IPCC AR6 WGII report[36] on the impact, adaptation, and vulnerability to climate change in part 6 and in their report[37] on how to mitigate climate change in part 7.
Download the bibliography here.
(IPCC, 2021) ↑
IPCC is an abbreviation for the Intergovernmental Panel on Climate Change, a U.N. agency. AR6 is their sixth major report on climate change, “Assessment Report 6.” ↑
There are several names for climate models, including atmosphere-ocean general circulation model (AOGCM, used in AR5), or Earth system model (ESM, used in AR6). Besides these complicated computer climate models there are other models used in AR6, some model energy flows, the impact of climate change on society or the global economy, or the impact of various greenhouse gas mitigation efforts. We only discuss some of these models in this report. (IPCC, 2021, p. 2223) ↑
(Manabe & Bryan, Climate Calculations with a Combined Ocean-Atmosphere Model, 1969), (Manabe & Wetherald, The Effects of Doubling the CO2 Concentration on the Climate of a General Circulation Model, 1975) ↑
(McKitrick & Christy, A Test of the Tropical 200- to 300-hPa Warming Rate in Climate Models, Earth and Space Science, 2018) and (McKitrick & Christy, 2020) ↑
(Box, 1976) ↑
Called petrophysical models. ↑
(Box, 1976) ↑
(IPCC, 1990) ↑
“The Intergovernmental Panel on Climate Change (IPCC) assesses the scientific, technical and socioeconomic information relevant for the understanding of the risk of human-induced climate change.” (UNFCCC, 2020). ↑
Usually, ECS means equilibrium climate sensitivity, or the ultimate change in surface temperature due to a doubling of CO2. but in AR6 sometimes they refer to “Effective Climate Sensitivity,” or the “effective ECS” which is defined as the warming after a specified number of years (IPCC, 2021, pp. 931-933). AR6, WGI, page 933 has a more complete definition. ↑
(Charney, et al., 1979) ↑
(Box, 1976) ↑
See https://www.ipcc.ch/reports/ ↑
CMIP5 and CMIP6 are the models used in AR5 and AR6 IPCC reports, respectively. ↑
(Eade, Stephenson, & Scaife, 2022) ↑
(Eade, Stephenson, & Scaife, 2022) ↑
(May, Is AR6 the worst and most biased IPCC Report?, 2023c; May, The IPCC AR6 Report Erases the Holocene, 2023d) ↑
(Crok & May, 2023, pp. 170-172) ↑
AR6, page 67. ↑
(Crok & May, 2023, pp. 108-113) ↑
(Crok & May, 2023, pp. 118-126) ↑
(Crok & May, 2023, pp. 140-149) ↑
Confirmation bias: The tendency to look only for data that supports a previously held belief. It also means all new data is interpreted in a way that supports a prior belief. Wikipedia has a fairly good article on common cognitive biases. ↑
Reporting bias: In this context it means only reporting or publishing results that favor a previously held belief and censoring or ignoring results that show the belief is questionable. ↑
The Dunning-Kruger effect is the tendency to overestimate one’s abilities in a particular subject. In this context we see climate modelers, who call themselves “climate scientists,” overestimate their knowledge of paleoclimatology, atmospheric sciences, and atomic physics. ↑
In-group bias causes lead authors and editors to choose their authors and research papers from their associates and friends who share their beliefs. ↑
Anchoring bias occurs when an early result or calculation, for example Svante Arrhenius’ ECS (climate sensitivity to CO2) of 4°C, discussed below, gets fixed in a researcher’s mind and then he “adjusts” his thinking and data interpretation to always come close to that value, while ignoring contrary data. ↑
(InterAcademy Council, 2010) ↑
(InterAcademy Council, 2010, pp. 17-18) ↑
(Crok & May, 2023) ↑
https://wcrp-cmip.org/cmip-phase-6-cmip6/ ↑
(IPCC, 2022) ↑
(IPCC, 2022b) ↑
Like this:
Loading…
Posted byAndy MayFebruary 28, 2024Posted inClimate models
Published by Andy May
61 NoTricksZone Articles On Studies, Datasets From 2023 Show Climate Models Are Rubbish
Dr Roy Spencer: New Global Dataset: Global Grids of UHI Effect On Air Temp, 1800-2023
2023/November/04/Dr Roy Spencer: New Global Dataset: Global Grids of UHI Effect On Air Temp, 1800-2023
Dr Roy Spencer: New Global Dataset: Global Grids of UHI Effect On Air Temp, 1800-2023
By P Gosselin on 4. November 2023
See full report at Dr. Roy Spencer
As a follow-on to our paper submitted on a new method for calculating the multi-station average urban heat island (UHI) effect on air temperature, I’ve extended that initial U.S.-based study of summertime UHI effects to global land areas in all seasons and produced a global gridded dataset, currently covering the period 1800 to 2023 (every 10 years from 1800 to 1950, then yearly after 1950).
It is based upon over 13 million station-pair measurements of inter-station differences in GHCN station temperatures and population density over the period 1880-2023.
Since UHI effects on air temperature are mostly at night, the results I get using Tavg will overestimate the UHI effect on daily high temperatures and underestimate the effect on daily low temperatures.
As an example of what one can do with the data, here is a global plot of the difference in July UHI warming between 1800 and 2023, where I have averaged the 1/12 deg spatial resolution data to 1/2 deg resolution for ease of plotting in Excel (I do not have a GIS system):
If I take the 100 locations with the largest amount of UHI warming between 1800 and 2023 and average their UHI temperatures together, I get the following:
Note that by 1800 there was 0.15 deg. C of average warming across these 100 cities since some of them are very old and already had large population densities by 1800. Also, these 100 “locations” are after averaging 1/12 deg. to 1/2 degree resolution, so each location is an average of 36 original resolution gridpoints. My point is that these are *large* heavily-urbanized locations, and the temperature signals would be stronger if I had used the 100 greatest UHI locations at original resolution.
Read entire article at Dr. Roy Spencer.
Solar forcing may have a 4 to 7 times greater effect on climate change than current climate models indicate
By Kenneth Richard on 5. October 2023
Share this…
Solar forcing may have a 4 to 7 times greater effect on climate change than current climate models indicate, which may mean modern climate change is predominantly natural rather than anthropogenic.
Anthropogenic global warming (AGW) attribution may be significantly dependent on the choice of dataset.
Advocates of AGW may only use Total Solar Irradiance (TSI) reconstructions that align with the perspective that the Sun has little to no impact on climate. Consequently, climate models may only use the PMOD’s model-based satellite data (which shows a declining trend since 1980) rather than the ACRIM (which shows an increasing TSI trend from the 1980s to 2000s).
The biased selection of long-term TSI reconstructions that show little to no variability are also preferred over TSI reconstructions with large variability. For example, the uncertainty in the estimate of the increase in TSI since the Maunder Minimum (1645-1715) ranges anywhere from 0.75 W/m² to 6.3 W/m² (Yeo et al., 2020). AGW advocates will, of course, select the lowest TSI change value (0.75 W/m²) and reject the higher values (6.3 W/m²), as then it is much easier to attribute modern warming to anthropogenic activity rather than to solar forcing.
The IPCC has selected one TSI dataset in the latest (2021) report for its global warming attribution assessments and climate models (GCMs). The dataset of course aligns only with the view modern warming is human-caused, and not natural, and thus it depicts a declining TSI trend since 1980 (PMOD) and almost no variability since the Maunder Minimum.
A new study (Connolly et al., 2023 with press release) identifies 27 other TSI estimates (purposely) ignored by the IPCC, several of which suggest modern warming may be up to 71-87% natural – especially if the temperature stations that do not show a strong artificial urban warming bias are used.
“Several of these different solar activity estimates suggest that most of the warming observed outside urban areas (in rural areas, oceans, and glaciers) could be explained in terms of the Sun.”
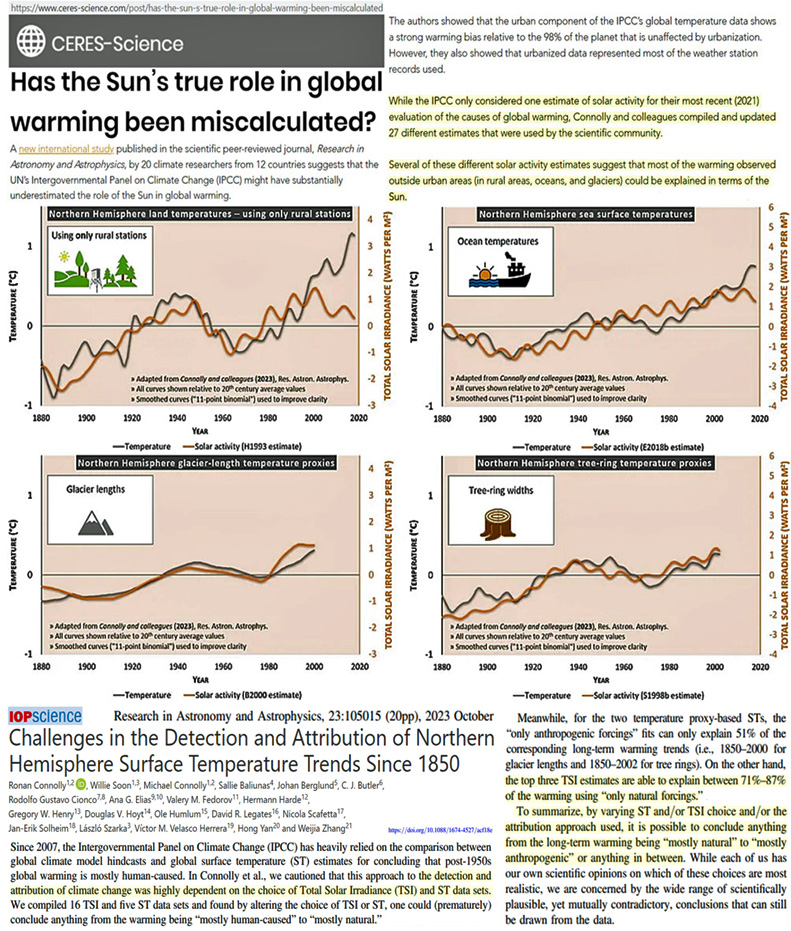
Image Source: Connolly et al., 2023 and press release
Another new study (Scafetta, 2023) suggests the Sun’s real climate impact may be 4-7 times larger than just from TSI (radiative) forcing alone, as the solar activity variations may mechanistically affect cloud albedo, which has been observed to drive 1-3 W/m² per decade changes in shortwave forcing (McLean, 2014).
“Thus, at least about 80% of the solar influence on the climate could be generated by processes other than direct TSI forcing.”
Climate models do not allow for any solar influence beyond the small, flat radiative forcing changes associated with TSI forcing, as this way it can be claimed that natural factors have little to no bearing on climate change.
Alternative solar activity records, as shown in TSI #2 Model below, have the Sun’s total impact directly linked to global temperature changes, including for recent decades.
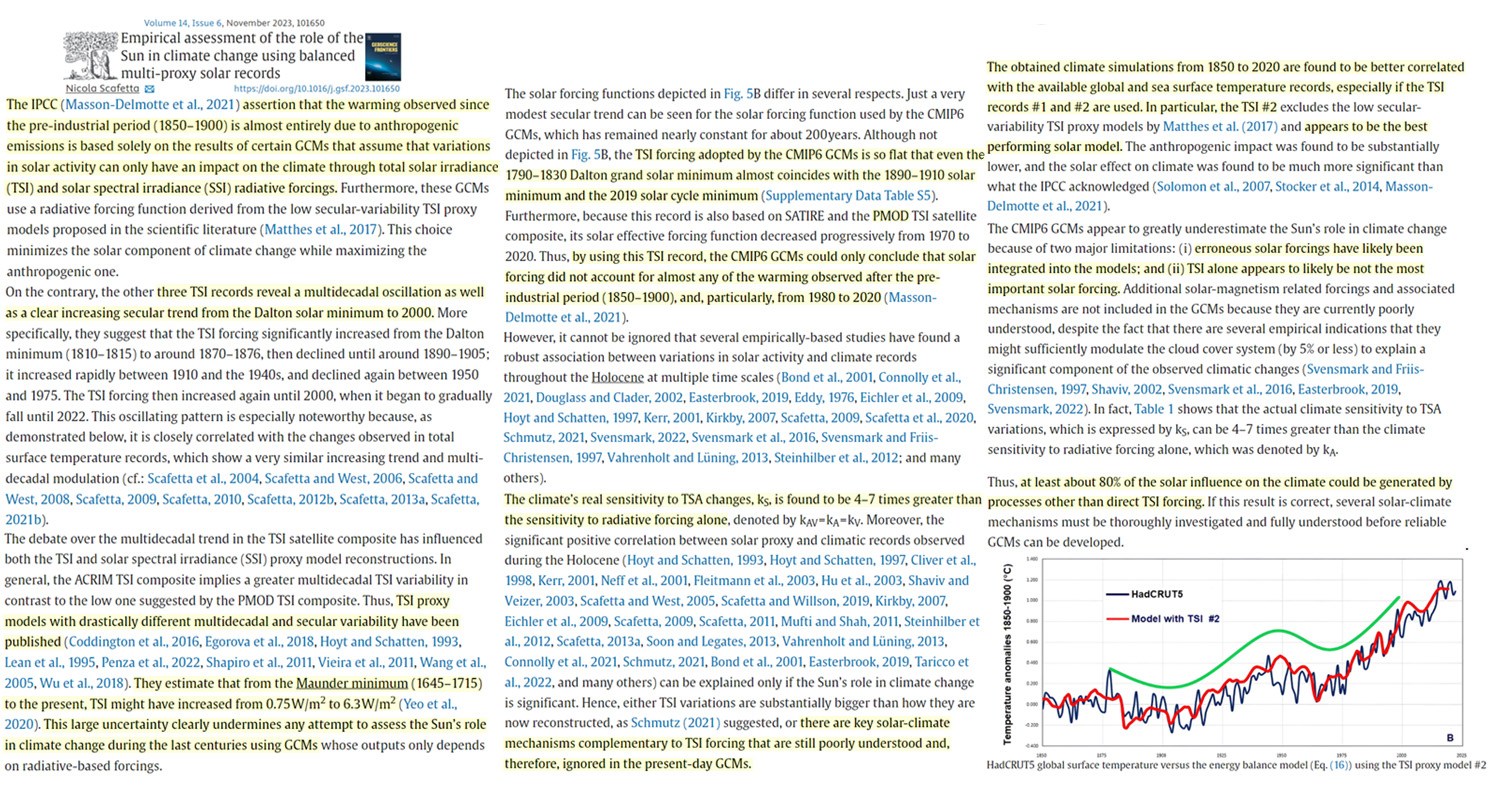
Image Source: Scafetta, 2023
Countless wind turbines…Northern Germany drought may in part be caused by efforts to prevent drought (climate change)!
3 months ago
Guest Blogger
From the NoTricksZone
By P Gosselin
Countless wind turbines…Northern Germany drought may in part be caused by efforts to prevent drought (climate change)!
More wind parks means less wind, which means less precipitation, which in turn means more drought and warmer temperatures.
Image: P. Gosselin
German online Reichschuster.de here reports on Gerd Ganteför, a German professor of experimental physics who taught at the University of Konstanz and Johns Hopkins University Baltimore (USA), among others. He has authored some 150 technical articles on renewable energies or climate change.
Ganteför has been an outspoken expert critic of Germany’s energy policy and the alarmist aspects of climate science.
Recently the renowned expert once again asked uncomfortable questions about possible connections between wind parks and their impact on regional climate. The answers Ganteför gave to the German daily “Nordkurier” have raised some eyebrows.
In summary, the physicist warns: “We don’t currently know what all can happen if we continue to put up countless wind turbines.”
The interview was prompted by a 2012 NASA study that suggested large wind farms in particular lead to an increase in the ambient temperature and are thus partly responsible for the warming of the climate.
Though Ganteför, has some doubts about this phenomenon, he nevertheless believes the “connection between wind turbines and global warming is possible – albeit for a reason not examined in the study,” reports Reichschuster.de “The authors were able to show that wind turbines swirl the cool layers of air that are directly above the ground and the somewhat warmer layers above them, and that this leads to an increase in temperature near the ground.”
Proven in other scientific publications
Ganteför, however, focusses on another aspect: evaporation, which has been proven in other publications.
The mechanism goes as follows: “Large wind turbines logically slow down the wind by sapping the energy out of it. Less wind means less evaporation and thus less precipitation. And if it gets drier, it could just happen that it gets warmer.”
A study of this kind by Deutsche Windguard was reported on by reitschuster.de in July 2022.
Overdoing wind energy
Moist air from the North Atlantic plays a major role on Europe’s climate, and eventually makes its way over the sea to Germany. But that air gets slowed down by the relatively large wind farms in Mecklenburg-Western Pomerania, says Ganteför. The possible consequence: “If you overdo it with too many wind turbines”, the region “will become drier” and “this possible scenario needs to be meticulously played out and studied by climatologists.”
“We don’t know at the moment what all can happen if we continue to put up countless wind turbines,” warns Ganteför.
New studies warn
Germany has so far installed over 30,000 wind turbines, which is about 1 every 11 sq. km. Plans are calling for doubling or even tripling the current wind power capacity. But this may be detrimental as new studies show that wind farms are altering local climates, and thus may be having an effect on global climate and contributing to regional droughts. We reported on this here earlier this month.
The “Green Energy”Duck Curve Threatens Grid Collapse
Have you heard of the duck curve? When Solar Power & Wind kick in, suddenly fossil generation must cease and when solar & wind collapse, fossil machines must suddenly generate putting enormous stresses on the machines.
Letter to EPA giving facts of CO2 & Global Warming
This is a great summary paper by the esteemed Authors William Happer & Richard Lindzen